The North Star Metric has become a staple in product strategy. It promises clarity, alignment, and a unified focus across teams. In theory, it’s the one metric that shows whether your customers are receiving value and whether your business is growing as a result.
But here’s the catch: most teams don’t stop to question what’s behind the number.
They see the chart and chase the trendline, but rarely ask, “Where is this coming from? What’s influencing the rise or fall? Can we trust it?”
If a North Star Metric can’t be explained or explored, it’s just a number on a screen – not a source of truth.
What Makes a North Star Metric Useful?
At its core, your North Star Metric should reflect how well you’re delivering value to your users. It offers a shared goal that connects departments; whether you’re in product, marketing, customer success, or operations.
A strong North Star Metric has three key qualities:
- It reflects meaningful customer value
- It can be influenced by your team’s efforts
- It acts as a leading indicator of long-term growth
When those conditions are met, your North Star Metric becomes a powerful decision-making tool. It helps teams align priorities, measure impact, and move forward together.
The Real Problem: You Can’t Act on What You Can’t See
Many teams adopt a North Star Metric because they’ve been told it’s a best practice. They choose a number, display it on a dashboard, and assume it will guide decisions.
But what happens when it stalls, spikes unexpectedly, or starts to lose relevance as the product evolves?
In many cases, no one knows the reason behind the change.
This is what we call the Visibility Gap – the disconnect between a company’s most important metric and their ability to understand or investigate it.
When a metric is divorced from its source, it loses its influence. Teams begin to question its accuracy. Decisions slow down. Alignment breaks down.
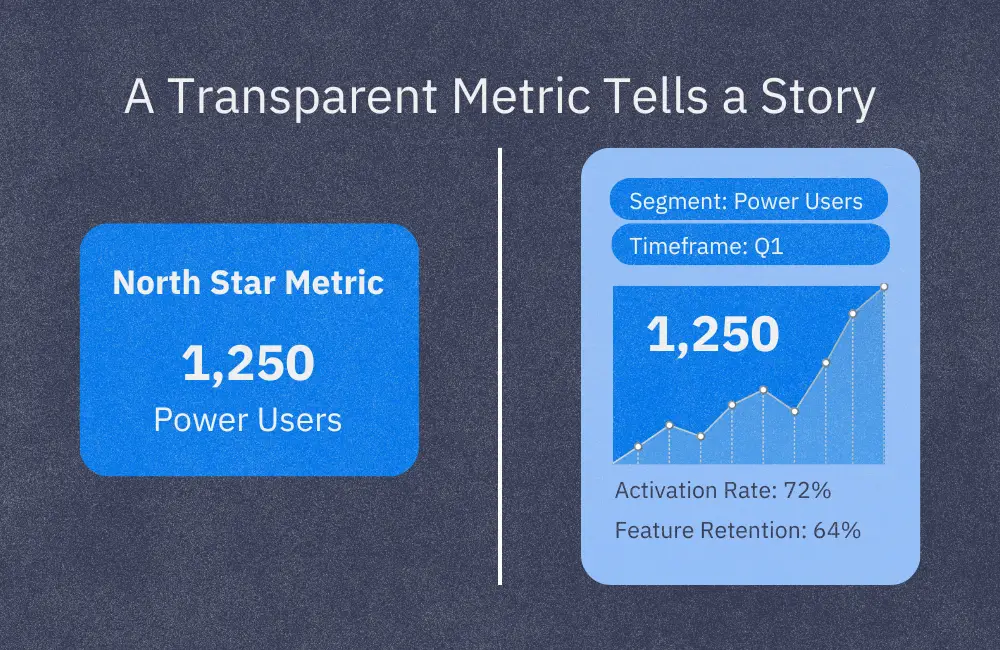
What Visibility Really Means
Visibility isn’t just about displaying a number. It’s about being able to trace its meaning and origins.
A transparent North Star Metric empowers your team to:
- Understand the logic and data sources behind the metric
- Break it down by customer segment, behavior, or timeframe
- Explore patterns or anomalies without relying solely on analysts
- Identify early signs of change in the supporting data
With this level of insight, your team can shift from reactive reporting to proactive strategy.
A New Way to Think About Your North Star
Rather than treating your North Star Metric as a static headline number, consider it a living reflection of customer value. It should evolve as your product grows and your users’ needs shift.
Supporting metrics, like activation rates, feature-level retention, or onboarding completion, add depth to your understanding. They don’t replace the North Star, but they provide the necessary context to act on it with confidence.
These metrics matter most when they’re clearly tied to your core metric and easy to access across teams. When both the primary and supporting metrics are transparent, teams gain more than visibility. They gain momentum.
Take a quick walkthrough of Kubit to see how teams explore their North Star Metric, and everything behind it, without writing a single line of SQL.
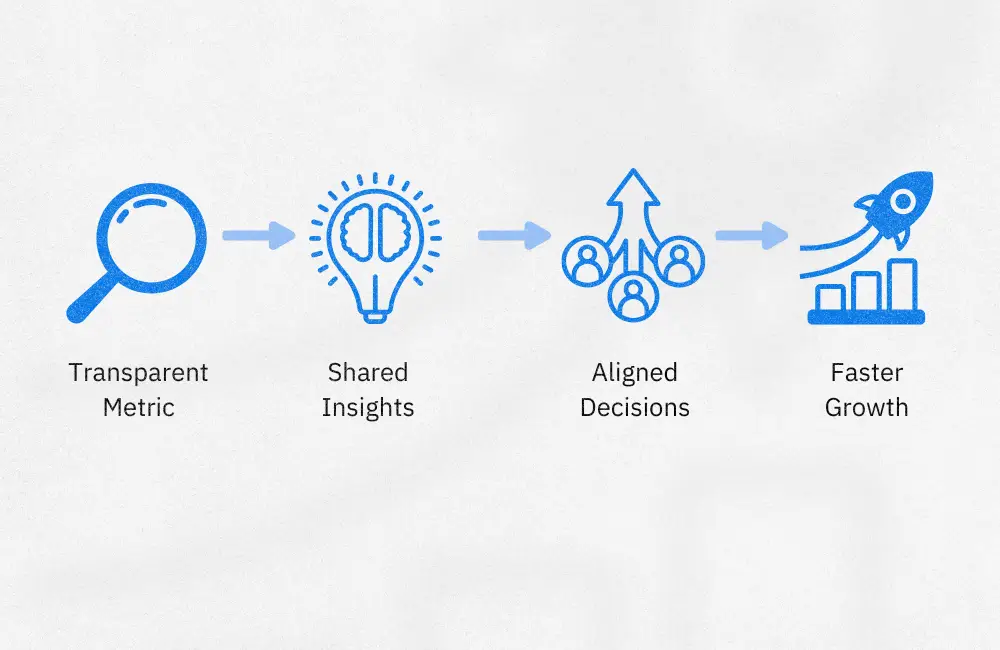
You Can’t Align Without Trust
Alignment is easy when everything is going well. But the real test comes when metrics change, priorities shift, or teams need to pivot quickly.
That’s when clarity matters most. And clarity depends on having data that is accessible, trusted, and easy to explore.
If your North Star Metric feels more like a mystery than a compass, it might be time to take a step back. Revisit what it’s measuring. Reassess how it’s defined. And ask whether your team has what they need to trust it.
Because a great metric isn’t just something you track. It’s something your entire team should understand and use every day.
Want to see what full transparency looks like?
Let’s talk about how Kubit can bring your North Star Metric into sharper focus—no black boxes, no delays, no guesswork.