One of the first questions I’ve seen asked in those big meetings, the ones we’ve all spent weeks or even months preparing for, is this: “Are these numbers accurate, or more directional?”
You break into a cold sweat…
I think they’re accurate?…
I think we used the same data from our main source of truth?…
Then, you nod in full confidence. “These are more… directional… For now.”
What a relief. Weight lifted off. Now, the meeting can continue.
But something changed, without anyone saying a word.
A giant shadow has fallen over the meeting. You realize that future facts and figures you present may be seen with that shadow hanging overhead.
Result: you didn’t make the impact you’d hoped for.
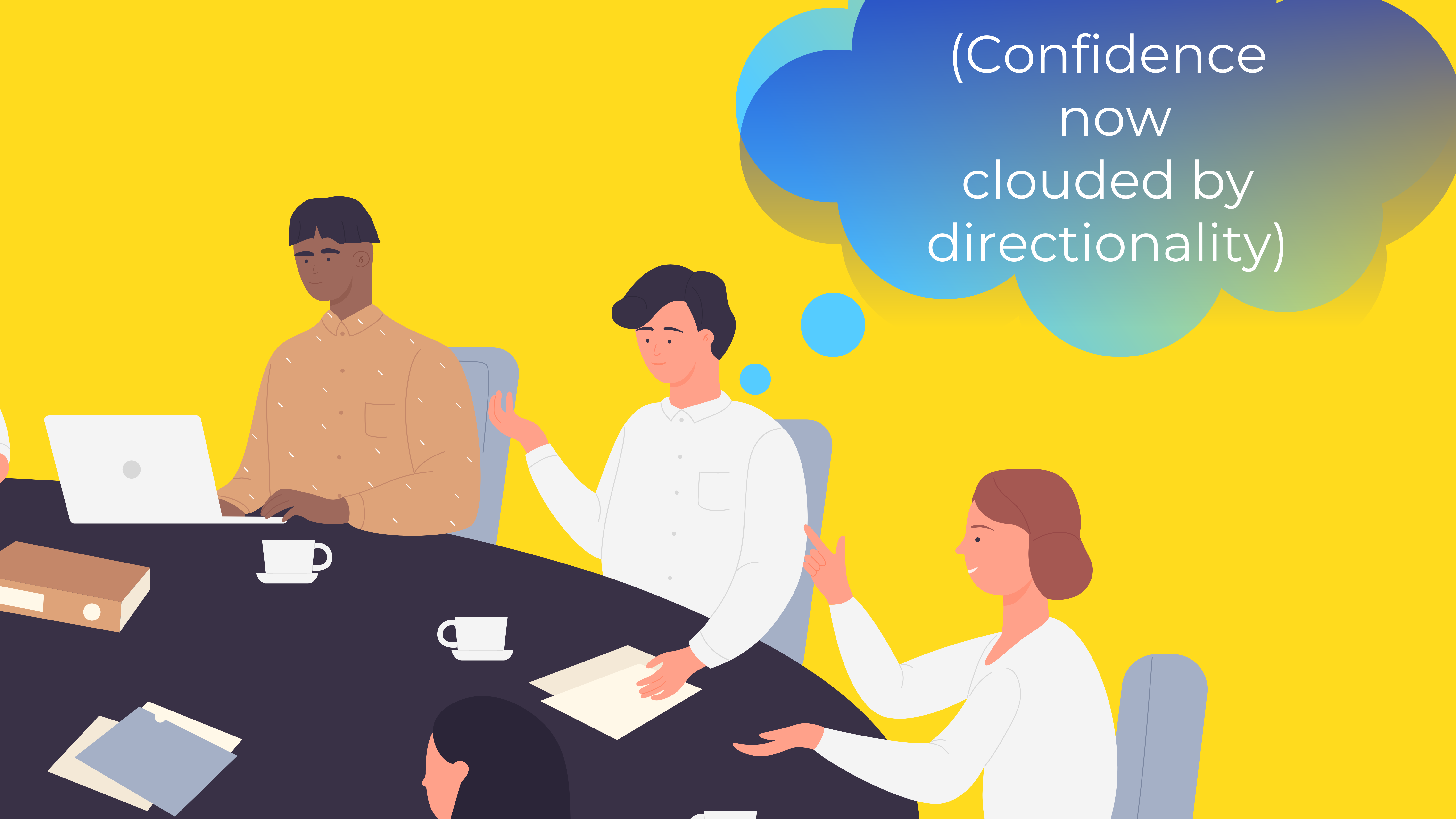
The tradeoff between numbers being accurate vs. being directional is an ongoing battle – and one that’s particularly challenging in Product Analytics, for reasons I’m going to explore in this article.
But first, a quick reminder of what we mean when we say “accurate” vs. “directional.”
“Accuracy” is when the information derived from your data can be confidently shared with internal and external stakeholders; it’s been “blessed” as delivered from your Single Source of Truth.
“Directional,” by contrast, refers to information that’s considered “good enough” to validate or justify initial decisions. Generally, directional data is not good enough to form the basis of the final numbers you present. And it certainly should not be part of the measurable outcomes shared with your stakeholders.
Often, the outcome of the accuracy-vs.-directional struggle isn’t poor decision-making, but a lack of decision-making; if you’re unsure of the accuracy of your data, then the default is to not take any action based upon it.
But, in Product Analytics, sometimes the worst thing you can do is nothing. Product Managers (PMs) are often expected to make decisions quickly – decisions that can have a major impact on revenue and user engagement.
Why accuracy vs. directionality is challenging in Product Analytics
There are two reasons why Product Analytics is unique when it comes to accuracy-vs.-directionality.
First, Product has an ENORMOUS amount of data. I’m talking trillions of events per day in some cases.
For many organizations, this data is monstrous, ever-changing, and non-standard. This means that fitting their product data into existing solutions for data governance becomes very challenging. Example: “Active User definition at Company A does not equal Active User definition at Company B.”
The second reason why the accuracy-vs.-directionality question is tricky in Product Analytics: Product people are relatively new to data being a core part of their day-to-day work, compared to teams like Business Intelligence (BI) or even Marketing – which have always been front-and-center in the data game. Building confidence in the data they use and making decisions off of it can be challenging for PMs, especially when it comes to the accuracy-vs.-directional tradeoff.
(Secret third reason… I work for a company that specializes in Product Analytics, Kubit… We all know what we are doing here, right?? 😂)

Traditionally, Product Managers shared data internally, and sometimes weren’t asked for data at all because it was too cumbersome to wrangle; however the prophecy that Product would become the profit center is coming true across many industries. With that high visibility, Product’s key numbers–like Monthly Active Users (MAU), total downloads, activated users from free-to-paid, etc.–have risen to the highest level of importance, sitting alongside the dollars and cents. This is an amazing development.
But with higher visibility comes greater scrutiny.
In this new Product-first world, the de facto mode must be accuracy. PMs and Data Engineers can no longer rely on directional metrics.
So, how do you make accuracy your de facto mode?
Collecting information is step one, and there are several methods that businesses use to accomplish this. Each has their own tradeoff and nuance (a topic that we can dive into in another blog), but let’s run through the highlights.
Tools that have their own data collection methods tend to be inflexible, forcing you to conform your data into their schema. Maybe you’ve been farming the collection out to an auto-tracking tool, or you trust the logging done to monitor uptime as “events.” These collection methods can lead to data that’s potentially accurate, but that may be prone to misalignment with how YOUR business thinks about this data.
The only way a company can fully understand where and how its product data came to be: first-party collection. But even first-party collection can be challenging!
So what do you do?
You collect stuff, using whichever method you decide. You need this data to make decisions on your product bets, experiments, and growth strategies. My point of view aligns with what we’re seeing in the market today: an increasing awareness that product data must live inside an organization’s source-of-truth data warehouse
Typically, data warehouses and BI reports are designed, governed, and maintained to uphold a single source of truth. If we want Product Analytics data to hold the weight it deserves, then it too must live up to this standard.
So… Once your Product Analytics data is stored in the data warehouse, you have the ability to access it via multiple solutions, and you’ve achieved accuracy nirvana…right? Not so fast.
Now, you have to decide how you want to analyze this data. Tools available in the Product Analytics space typically follow the same value proposition: “send us your data, and we’ll optimize it for you so you can run high-performance queries on complex user journeys.” This is great! Until… certain limitations arise.
When your user base grows and events balloon, now you have to pay a large bill, or begin pruning that data via sampling or excluding events. Another problem: you’ve also created another “source of truth,” because the data has left the warehouse. When it breaks, who fixes it? Now, we’re creeping into directional territory…
Before you know it, you can see the directionality shadow encroaching into your next Product leadership meeting.
With next-gen tools, directional data can be a thing of the past
To avoid the accuracy-vs.-directionality tradeoff, a new generation of tools have emerged that leverage your data warehouse directly – no sampling or pruning needed, and no alternative “truth” sources created in silos. These new solutions provide insights using existing data investments made by your organization, leveraging the cloud and removing the requirement to ship data outside your firewalls. The result of this warehouse-native approach: you have Product Managers who are enabled to work with fully accurate data.
Rachel Herrera leads Customer Success at Kubit, the first warehouse-native Product Analytics platform. Do you have thoughts on accuracy-vs.-directionality or on improving your product analytics workflows or infrastructure? Drop her a note at rachel.herrera@kubit.co.